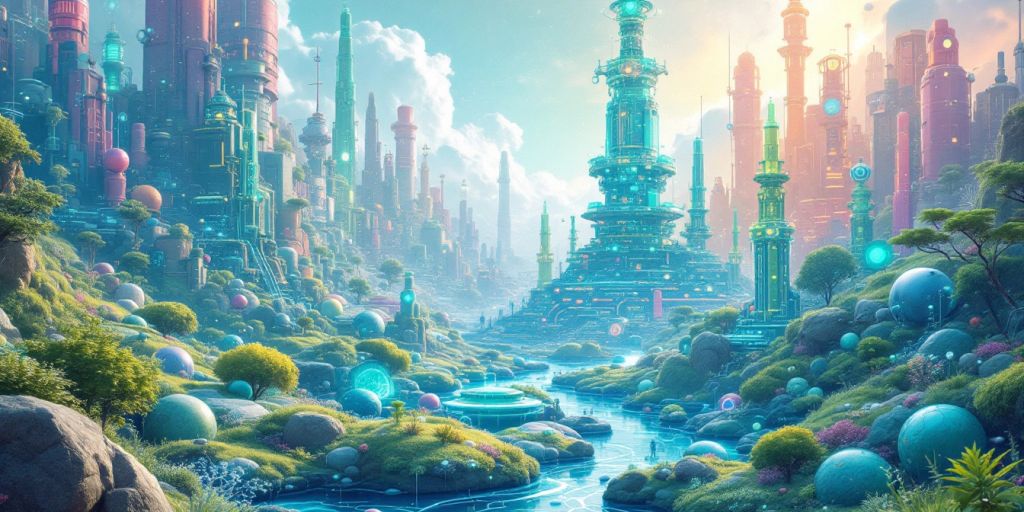
In this article, we will explore the exciting world of Reinforcement Learning (RL), a key area in Artificial Intelligence that allows machines to learn and improve through experiences. By understanding how RL works, its challenges, and its future directions, we can better appreciate its potential impact on various industries.
Key Takeaways
- Reinforcement Learning helps machines learn by trying out actions and getting feedback.
- Balancing exploration of new ideas with using known successful actions is a major challenge.
- RL can be used in many fields, including gaming, robotics, and healthcare.
- Future advancements may include combining RL with other AI techniques and improving learning efficiency.
- Ethical considerations are important to ensure fairness and prevent bias in AI systems.
Key Concepts in Reinforcement Learning
Reinforcement Learning (RL) is a unique area of artificial intelligence that focuses on how agents learn to make decisions through interactions with their environment. The key concepts of reinforcement learning include several important components:
Understanding Agents and Environments
- Agent: The learner or decision-maker that interacts with the environment.
- Environment: Everything the agent interacts with, including all possible states and outcomes.
- State: A specific situation in which the agent finds itself at any given time.
The Role of Rewards and Penalties
- Reward: Feedback from the environment that indicates how good or bad an action was.
- Penalty: Negative feedback that discourages certain actions.
- The agent’s goal is to maximize its total rewards over time by learning from these feedback signals.
Policy and Value Functions
- Policy: A strategy that the agent uses to decide which action to take in a given state.
- Value Function: A prediction of the expected reward for each state, helping the agent evaluate the long-term benefits of its actions.
In RL, learning occurs through trial and error, where agents adapt their strategies based on the outcomes of their actions. This process mirrors how humans learn from experiences.
Understanding these concepts is crucial for grasping how reinforcement learning operates and its potential applications in various fields.
Challenges in Reinforcement Learning
Reinforcement Learning (RL) is a powerful tool, but it comes with its own set of challenges that can make it difficult to implement effectively. Here are some of the main issues:
Exploration vs. Exploitation Dilemma
One of the biggest challenges in RL is the exploration vs. exploitation dilemma. Agents must decide whether to try new actions that might lead to better rewards (exploration) or stick with actions that have worked well in the past (exploitation). Finding the right balance is crucial for success.
Sample Efficiency Issues
Another significant challenge is sample efficiency. RL often requires a lot of interactions with the environment to learn effectively. This can be a problem in real-world situations where gathering data can be time-consuming or costly. Improving sample efficiency is essential for making RL practical in various applications.
Generalization to Unseen Environments
Lastly, RL struggles with generalization. Agents often find it hard to apply what they’ve learned in one situation to new, unseen environments. This limitation can hinder their performance and adaptability. To address this, researchers are developing methods to help agents transfer knowledge from one task to another.
The challenges in reinforcement learning highlight the need for ongoing research and innovation to make these systems more effective and applicable in real-world scenarios.
Challenge | Description |
---|---|
Exploration vs. Exploitation | Balancing trying new actions with using known rewarding actions. |
Sample Efficiency | The need for many interactions to learn effectively, which can be costly in real life. |
Generalization | Difficulty in applying learned knowledge to new situations. |
By understanding these challenges, we can better navigate the complexities of reinforcement learning and work towards more effective solutions.
Real-World Applications of Reinforcement Learning
Reinforcement Learning (RL) is making a big impact in many areas:
Advancements in Gaming
In gaming, RL is used to create smarter AI. It helps design non-playable characters (NPCs) that can adapt and strategize, making games more exciting and challenging for players.
Robotics and Automation
Robots are learning to perform tasks through RL. They can:
- Grasp objects
- Navigate through spaces
- Perform complex movements
This makes them more efficient and capable in unpredictable environments.
Healthcare Innovations
In healthcare, RL is being applied to improve patient care. It helps in:
- Personalized treatment plans
- Drug discovery
- Optimizing diagnosis
These advancements lead to better outcomes for patients.
Reinforcement Learning is transforming industries by enabling machines to learn from their experiences, making them smarter and more efficient.
Application Area | Key Benefits |
---|---|
Gaming | Smarter NPCs, enhanced player experience |
Robotics | Increased efficiency, adaptability |
Healthcare | Improved treatment, personalized care |
Autonomous Vehicles | Safer navigation, better decision-making |
Finance | Optimized trading strategies, risk management |
In summary, RL is not just a theoretical concept; it is actively shaping the future of various industries, making them more innovative and effective.
Future Directions in Reinforcement Learning
Deep Reinforcement Learning
Deep Reinforcement Learning (DRL) combines deep learning with reinforcement learning, allowing agents to handle complex tasks. This approach has led to significant advancements in various fields, enabling systems to learn from vast amounts of data. DRL has been particularly successful in gaming, where agents have outperformed human players in challenging games.
Transfer Learning
Transfer learning is a promising area that helps agents apply knowledge from one task to another. This can greatly reduce the time and resources needed for training. By leveraging previous experiences, agents can learn faster and adapt to new challenges more efficiently.
Multi-Agent Systems
In many real-world situations, multiple agents interact with each other. Research in multi-agent systems focuses on how these agents can cooperate or compete effectively. This could lead to breakthroughs in areas like traffic management and collaborative robotics.
Trend | Description | Potential Impact |
---|---|---|
Deep Reinforcement Learning | Combines deep learning with RL for complex tasks. | Enhanced performance in gaming and robotics. |
Transfer Learning | Allows agents to use knowledge from one task in another. | Faster training and adaptability. |
Multi-Agent Systems | Studies interactions between multiple agents. | Improved efficiency in real-world applications. |
As we look to the future, the integration of these advancements will likely reshape the landscape of artificial intelligence, making systems more adaptable and efficient. The potential for RL to revolutionize various industries is immense.
Ethical and Societal Implications
As artificial intelligence becomes more common, it’s important to think about the ethical issues that come with it. Reinforcement Learning (RL) is no different. Here are some key points to consider:
Ensuring Fairness and Avoiding Bias
- Fairness is crucial. We must ensure that RL systems do not discriminate against individuals or groups based on characteristics such as race, gender, or age.
- Developers should regularly check their algorithms for any signs of bias.
- Transparency in how decisions are made can help build trust.
Ethical Considerations in Autonomous Systems
- Autonomous systems, like self-driving cars, must be designed with ethics in mind.
- Decisions made by these systems can have serious consequences, so they need to be accountable.
- Stakeholders should engage in discussions about the moral implications of these technologies.
Impact on Employment and Society
- The rise of RL could change job markets significantly.
- While some jobs may be lost, new opportunities in AI and tech will emerge.
- Society must adapt to these changes, ensuring that everyone benefits from advancements in technology.
As we explore the future of AI, it’s essential to remain aware of the potential challenges and ethical considerations that accompany the development of powerful systems.
By addressing these ethical and societal implications, we can work towards a future where AI benefits everyone, promoting fairness and accountability in its applications.
Integrating Reinforcement Learning with Other AI Techniques
Reinforcement Learning (RL) is not just a standalone technique; it can be combined with other AI methods to create more powerful systems. Here are some ways RL integrates with other AI techniques:
Combining with Natural Language Processing
- Enhanced Communication: RL can improve how chatbots understand and respond to users.
- Learning from Feedback: Chatbots can learn from user interactions to provide better answers over time.
- Dynamic Responses: RL helps in adapting responses based on the context of the conversation.
Enhancing Computer Vision
- Object Recognition: RL can help systems learn to identify objects in images more accurately.
- Real-Time Decision Making: In robotics, RL allows machines to make quick decisions based on visual input.
- Improved Tracking: RL can enhance the ability to track moving objects in video feeds.
Synergies with Supervised and Unsupervised Learning
- Data Efficiency: RL can use data from supervised learning to speed up its training process.
- Feature Learning: Unsupervised learning can help RL agents identify important features in their environment.
- Robust Models: Combining these techniques can lead to more robust AI models that perform better in real-world scenarios.
Integrating RL with other AI techniques opens up new possibilities, making systems smarter and more adaptable.
By exploring these integrations, we can unlock the full potential of AI, leading to exciting advancements in various fields.
Case Studies in Reinforcement Learning
Reinforcement Learning (RL) has shown its potential through various real-world applications. Here are some notable case studies:
Self-Driving Vehicles
Self-driving cars use RL to learn how to navigate through traffic. The car acts as an agent, making decisions like when to stop or turn. By receiving rewards for safe driving and penalties for mistakes, these vehicles improve their driving skills over time.
Automated Financial Trading
In finance, RL is used to create trading systems that can buy and sell stocks automatically. These systems learn from market changes and adjust their strategies to maximize profits. Here’s how it works:
- The agent observes market conditions.
- It decides whether to buy, sell, or hold.
- It receives feedback based on the outcome of its actions.
Enhancing Cloud Deployments
Companies use RL to manage resources in cloud computing. By optimizing how resources are allocated, businesses can save money and improve performance. The RL agent learns to make decisions that balance cost and efficiency.
Reinforcement Learning is not just a theoretical concept; it is actively shaping industries and improving technologies.
These case studies highlight how RL is transforming various fields, making processes smarter and more efficient.
Conclusion
In conclusion, Reinforcement Learning (RL) is changing the way we think about artificial intelligence. It allows machines to learn from their experiences, much like how we learn from our mistakes and successes. Although there are challenges to overcome, such as how to balance trying new things with using what we already know, the future looks bright. As researchers continue to improve RL techniques, we can expect to see it making a big impact in many areas, including gaming, healthcare, and finance. The journey of RL is just beginning, and its potential to transform our world is exciting.
Frequently Asked Questions
What is Reinforcement Learning?
Reinforcement Learning (RL) is a way for computers to learn how to make decisions by trying things out and seeing what happens. It’s like when you learn from your mistakes and successes.
How does an agent learn in Reinforcement Learning?
An agent learns by getting rewards for good actions and penalties for bad ones, helping it figure out the best things to do in different situations.
What are some challenges in Reinforcement Learning?
Some challenges include deciding when to try new things versus using what you already know, needing lots of examples to learn, and making sure it can work well in new situations.
Where is Reinforcement Learning used in real life?
Reinforcement Learning is used in many areas like video games, robots, self-driving cars, and even in healthcare for better treatment plans.
What does the future hold for Reinforcement Learning?
The future of Reinforcement Learning looks bright with new ideas like using deep learning, sharing knowledge between tasks, and having multiple agents work together.
Why is ethics important in Reinforcement Learning?
Ethics is important because as AI systems make decisions, we need to ensure they are fair and do not cause harm to people or society.