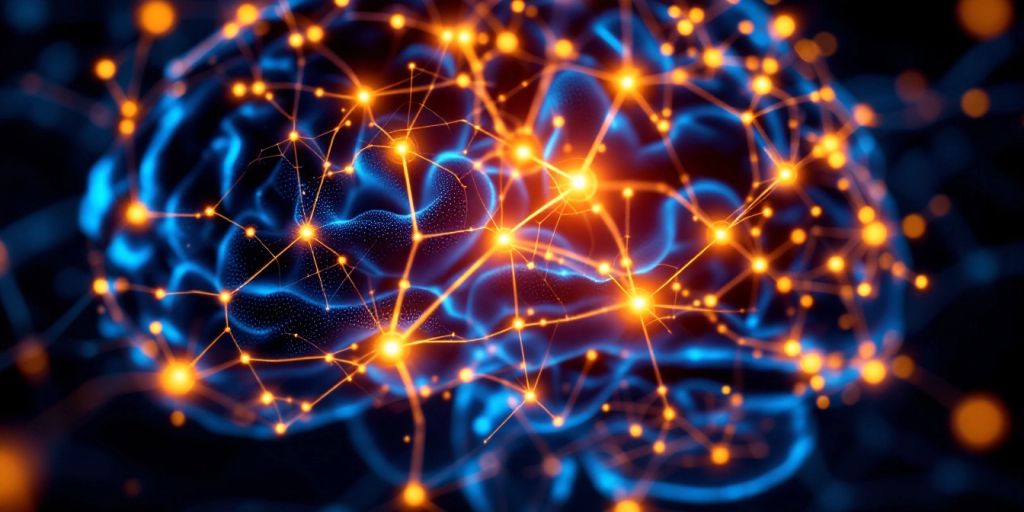
Chain-of-thought prompting is a fresh take on how artificial intelligence (AI) can think and solve problems. By breaking down tough questions into smaller, easier parts, AI can reason more like humans. This method helps AI explain its thinking clearly, making it easier for people to understand. In this article, we will explore what chain-of-thought prompting is, how it works, where it’s used, and what its future might hold.
Key Takeaways
- Chain-of-thought prompting helps AI break down complex problems into simpler steps.
- This method makes AI reasoning clearer and easier to follow for users.
- Research shows that using chain-of-thought can improve AI performance in areas like education and healthcare.
- While beneficial, there are challenges such as the need for more computing power and the risk of confusing reasoning.
- The future of AI may include even better ways to use chain-of-thought prompting, making AI smarter and more transparent.
Understanding Chain-of-Thought Prompting
Definition and Concept
Chain-of-Thought (CoT) prompting is a method that helps AI systems think more clearly by breaking down complex problems into smaller, manageable steps. This approach allows AI to provide better answers by mimicking how humans reason through problems. Instead of trying to solve everything at once, CoT prompting encourages a step-by-step process, making it easier for the AI to understand and respond accurately.
Historical Background
The idea of CoT prompting comes from studies in psychology about how people solve problems. Researchers noticed that when humans think aloud or verbalize their thoughts, they can tackle complex issues more effectively. This led to the development of CoT prompting in AI, aiming to improve how AI models handle difficult tasks by guiding them through logical reasoning steps.
Core Principles of CoT
The main principles of Chain-of-Thought prompting include:
- Sequential Reasoning: Breaking down problems into a series of steps.
- Clarity: Providing clear instructions to help the AI understand the task.
- Transparency: Making the AI’s thought process easier to follow, which helps users trust its responses.
By using Chain-of-Thought prompting, AI can tackle complex challenges more like humans do, leading to better outcomes and understanding.
Methodologies in Chain-of-Thought Prompting
Example-Based Learning
Example-based learning is a key method in Chain-of-Thought (CoT) prompting. In this approach, the AI is shown specific examples that illustrate how to break down complex problems into simpler steps. This helps the AI understand the reasoning process better. Here are some key points about this method:
- It provides clear examples of how to approach a problem.
- The AI learns to replicate the reasoning shown in the examples.
- It enhances the AI’s ability to tackle new problems effectively.
Step-by-Step Articulation
In this methodology, the AI is encouraged to think through problems step by step. This means that instead of jumping to conclusions, the AI lays out its reasoning in a clear sequence. The process typically involves:
- Identifying the problem clearly.
- Breaking it down into smaller, manageable parts.
- Explaining each part before arriving at a final answer.
This structured approach mirrors how humans solve problems, making the AI’s reasoning more transparent.
Final Answer Generation
After articulating its thoughts, the AI generates a final answer based on the reasoning steps it has outlined. This final step is crucial as it combines all the previous steps into a coherent solution. The benefits of this method include:
- Improved accuracy in answers.
- Enhanced clarity in the AI’s thought process.
- Better understanding of complex issues.
By using these methodologies, Chain-of-Thought prompting significantly boosts the AI’s problem-solving abilities, making it a powerful tool in various applications.
Applications of Chain-of-Thought in Various Fields
Medical Diagnosis
Chain-of-thought prompting is making a significant impact in the medical field. AI systems can now provide clearer explanations for their diagnoses. For example, an AI might say, "Given the patient’s symptoms and test results, I suspect appendicitis because…" This transparency helps doctors and patients understand the reasoning behind medical decisions.
Educational Tools
In education, CoT is transforming how students learn. AI tutors can break down complex problems into simpler steps, making it easier for students to grasp difficult concepts. Research shows that students using CoT-enhanced tutoring systems perform better in subjects like math. Here are some benefits:
- Improved understanding of complex topics
- Increased student engagement
- Higher overall academic performance
Customer Support Systems
CoT is also enhancing customer support. Chatbots that use this method can provide more accurate and relevant answers. By following a logical sequence, they can better understand customer queries and respond effectively. This leads to:
- Faster resolution of issues
- Higher customer satisfaction
- More personalized interactions
Summary Table of Applications
Field | Benefits of CoT Prompting |
---|---|
Medical Diagnosis | Clearer explanations for diagnoses |
Educational Tools | Improved understanding and engagement |
Customer Support | Faster issue resolution and personalized service |
Chain-of-thought prompting is reshaping how AI interacts with various fields, making it a powerful tool for enhancing understanding and decision-making.
Advantages and Limitations of Chain-of-Thought
Enhanced Problem-Solving
Chain-of-thought (CoT) prompting significantly boosts AI’s ability to tackle complex problems. By breaking down tasks into smaller steps, AI can analyze and solve issues more effectively. This method allows for a clearer understanding of the reasoning process, making it easier for users to follow along.
Transparency in AI Reasoning
One of the key benefits of CoT is that it makes AI’s decision-making process more transparent. Users can see how the AI arrives at its conclusions, which builds trust and confidence in its outputs. This transparency is crucial in fields like healthcare, where understanding the reasoning behind a diagnosis can be vital.
Challenges and Limitations
Despite its advantages, CoT prompting has some limitations:
- Zero-Shot Reasoning: While AI can perform well in zero-shot scenarios, it can still make mistakes in its reasoning chains. This can happen due to a lack of understanding of context or nuances.
- Manual Effort Required: Creating effective CoT prompts often requires significant human input, which can be time-consuming.
- Automatic Prompting Issues: Ensuring that AI-generated explanations are accurate and human-like remains a challenge. Crafting effective prompts requires a deep understanding of language models.
Advantages | Limitations |
---|---|
Enhanced problem-solving | Zero-shot reasoning errors |
Transparency in reasoning | Manual effort needed for prompts |
Improved content quality | Challenges in automatic prompting |
CoT prompting is a powerful tool that enhances AI’s reasoning capabilities, but it is essential to be aware of its limitations to use it effectively.
Future Prospects of Chain-of-Thought
Advancements in AI Reasoning
The future of Chain-of-Thought (CoT) prompting looks bright. As AI technology evolves, CoT will play a crucial role in enhancing reasoning capabilities. This could lead to:
- More complex problem-solving abilities.
- Improved understanding of human-like reasoning.
- Greater transparency in AI decision-making.
Potential in Emerging Technologies
CoT prompting is expected to find applications in various new technologies, such as:
- Healthcare: AI could provide clearer explanations for diagnoses, making it easier for doctors and patients to understand.
- Education: AI tutors may become more effective by breaking down complex subjects into simpler parts.
- Customer Support: AI systems could offer better assistance by explaining their reasoning behind solutions.
Impact on AI Transparency
The rise of CoT prompting is changing how we view AI. It emphasizes making AI’s thought processes clearer and more understandable. This shift could lead to:
- Increased trust in AI systems.
- Better collaboration between humans and AI.
- Enhanced user experience across various applications.
The evolution of Chain-of-Thought prompting is not just about improving AI; it’s about making AI more relatable and understandable for everyone.
Case Studies and Real-World Implementations
Stanford University Research
Researchers at Stanford University are investigating how Chain-of-Thought (CoT) can enhance medical AI systems. This approach aims to make AI more transparent, allowing it to explain its reasoning. For example, an AI might say, "Given the patient’s elevated white blood cell count and localized pain, I suspect appendicitis. Here’s why…" This clarity can help both doctors and patients understand the diagnosis better.
Carnegie Mellon University Study
A study from Carnegie Mellon University shows that CoT can significantly improve student learning outcomes in mathematics. By breaking down complex problems into simpler steps, AI tutors can guide students more effectively. This method transforms AI from a strict taskmaster into a patient explainer, making learning more engaging.
DeepMind’s Tree of Thoughts
DeepMind has developed a technique called "Tree of Thoughts," which allows AI to explore multiple reasoning paths at once. This innovation enables AI to brainstorm and consider various solutions simultaneously, enhancing its problem-solving capabilities. It’s like giving AI the ability to think creatively, rather than just following a single line of reasoning.
In summary, these case studies illustrate the power of Chain-of-Thought in real-world applications, showcasing its potential to improve transparency, education, and creative problem-solving in AI systems.
Techniques to Improve Chain-of-Thought Prompting
Manual vs. Automatic CoT
To enhance the effectiveness of chain-of-thought (CoT) prompting, two main techniques can be utilized:
- Manual CoT: This involves carefully crafting prompts that guide the AI through a series of logical steps. For example, when solving a math problem, the prompt might instruct the AI to first identify the numbers involved, then perform calculations step-by-step.
- Automatic CoT: This method automates the process of generating reasoning chains. By using algorithms, the AI can create its own prompts based on previous examples, making it more adaptable to various tasks.
Improving Prompt Designs
Creating effective prompts is crucial for successful CoT prompting. Here are some tips:
- Be Clear: Use simple language and clear instructions.
- Use Examples: Provide examples that illustrate the desired reasoning process.
- Encourage Step-by-Step Thinking: Phrases like "Let’s think step by step" can prompt the AI to articulate its thought process.
Ensuring Accuracy in Reasoning
To maintain high accuracy in AI responses, consider the following:
- Review Outputs: Regularly check the AI’s answers for correctness.
- Refine Prompts: Adjust prompts based on the AI’s performance to improve clarity and effectiveness.
- Feedback Loop: Implement a system where the AI learns from its mistakes to enhance future reasoning.
By focusing on these techniques, we can significantly enhance the AI’s reasoning capabilities, making it more effective in solving complex problems.
Technique | Description |
---|---|
Manual CoT | Crafting prompts that guide AI through logical steps. |
Automatic CoT | Using algorithms to generate reasoning chains automatically. |
Improving Prompt Designs | Creating clear, example-driven prompts that encourage step-by-step thinking. |
Conclusion
In summary, chain-of-thought prompting is a big step forward in artificial intelligence. It helps AI think more clearly and solve problems better by breaking down complex tasks into simpler steps. This method not only makes AI’s reasoning easier to understand but also boosts its performance in various fields like education, healthcare, and finance. As we continue to explore and improve this technique, the future looks bright for AI, making it more reliable and effective in real-world situations.
Frequently Asked Questions
What is Chain-of-Thought prompting?
Chain-of-Thought (CoT) prompting is a method that helps AI break down complex problems into simpler steps. This makes it easier for AI to solve tough questions and explain its reasoning.
How does Chain-of-Thought prompting work?
It works by guiding AI to think step by step. Instead of jumping to an answer, AI is encouraged to explain its thought process, which helps it arrive at a more accurate conclusion.
What are some benefits of using Chain-of-Thought prompting?
Some benefits include better problem-solving abilities, clearer explanations from AI, and improved understanding of AI’s reasoning, which can help in various fields like education and healthcare.
Are there any challenges with Chain-of-Thought prompting?
Yes, there are challenges. For example, it can require a lot of computer power, and sometimes AI might still make mistakes in its reasoning.
Where is Chain-of-Thought prompting being used?
It’s being used in many areas, including medical diagnostics, educational tools, and customer support systems, to make AI more effective and transparent.
What does the future hold for Chain-of-Thought prompting?
The future looks promising! As AI technology improves, we can expect even better ways to design prompts that help AI think more like humans, leading to more accurate and understandable results.