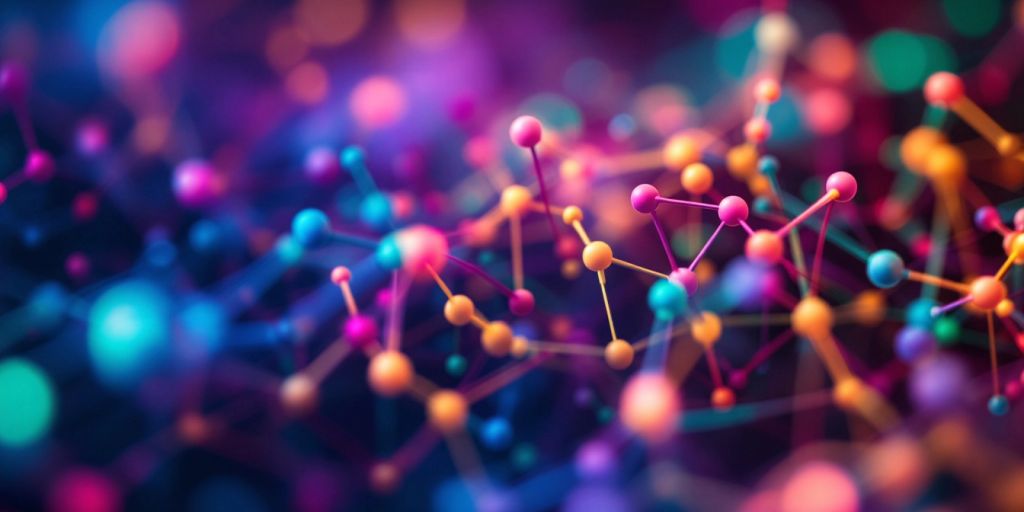
Artificial intelligence (AI) is transforming many fields, but one major issue it faces is the problem of hallucinations. Hallucinations occur when AI systems generate false or misleading information. This article explores innovative methods to reduce these inaccuracies, ensuring AI can be more reliable and trustworthy. By understanding the causes of hallucinations and implementing effective techniques, we can improve AI’s performance and reliability across various applications.
Key Takeaways
- AI hallucinations refer to instances where AI provides incorrect or misleading information.
- Common reasons for hallucinations include poor training data and biases in algorithms.
- Techniques like predictive measurement and semantic checks can help identify and reduce hallucinations.
- Retrieval-Augmented Generation (RAG) can enhance AI responses by grounding them in reliable data.
- Regular monitoring and updating of AI systems are essential for maintaining accuracy.
Understanding AI Hallucinations
Defining Hallucinations in AI
AI hallucinations refer to instances when artificial intelligence systems generate information that is incorrect or misleading. These errors can mislead users and undermine trust in AI technologies. Hallucinations can occur in various forms, including:
- Confabulations: Providing inconsistent wrong answers.
- Fabrications: Creating entirely false information.
- Inaccurate Assertions: Presenting incorrect facts confidently.
Common Causes of AI Hallucinations
Several factors contribute to AI hallucinations, including:
- Training Data Quality: Poor or biased data can lead to incorrect outputs.
- Model Complexity: More complex models may struggle to maintain accuracy.
- Lack of Context: AI may not fully understand the context of a query, leading to errors.
Impact of Hallucinations on AI Reliability
The presence of hallucinations can significantly affect the reliability of AI systems. For instance:
- Users may lose trust in AI tools.
- Businesses could face legal issues due to misinformation.
- Hallucinations can lead to costly mistakes, especially in critical fields like healthcare or law.
Hallucinations in AI are not just minor errors; they can have serious consequences, especially in high-stakes environments.
In summary, understanding AI hallucinations is crucial for improving the reliability of these systems. By addressing the causes and impacts, we can work towards reducing their occurrence and enhancing user trust.
Techniques for Reducing AI Hallucinations
Predictive Hallucination Measurement
One effective way to tackle AI hallucinations is through predictive hallucination measurement. This method acts like a benchmark exam for AI systems, helping to identify when they might produce incorrect information. By analyzing past outputs, we can predict future errors and improve reliability.
Dimensionality Reduction
Another innovative technique is dimensionality reduction. This process simplifies complex data into fewer dimensions, making it easier to spot inaccuracies. For example, reducing a model’s data from 1,536 dimensions to just three can help separate correct responses from hallucinations with about 80% accuracy. This method allows us to focus on the most important data, filtering out noise that could lead to errors.
Semantic Entropy Detection
Lastly, semantic entropy detection helps identify when an AI is likely to hallucinate by measuring the uncertainty in its responses. If an AI’s answer has high entropy, it may indicate a lack of confidence, suggesting that the information could be unreliable. By monitoring these signals, we can take steps to improve the AI’s output.
Understanding these techniques is crucial for developing more reliable AI systems. By implementing these methods, we can significantly reduce the chances of AI hallucinations and enhance overall performance.
These techniques are essential for ensuring that AI systems provide accurate and trustworthy information, ultimately leading to better user experiences and outcomes.
Role of Training Data in Hallucination Reduction
Importance of High-Quality Training Data
High-quality training data is essential for reducing hallucinations in AI systems. When the data is accurate and reliable, the AI is more likely to produce correct outputs. Here are some key points:
- High-quality data helps the AI learn better.
- It reduces the chances of the AI making mistakes.
- Regular updates to the data keep it relevant.
Biases in Training Data
Biases in training data can lead to incorrect outputs. If the data is skewed or unbalanced, the AI may produce biased or inaccurate results. Here are some common biases:
- Selection Bias: Only certain types of data are included.
- Label Bias: Incorrect labels can mislead the AI.
- Confirmation Bias: Data that supports existing beliefs is favored.
Updating Training Data Regularly
Regularly updating training data is crucial for maintaining AI accuracy. This process involves:
- Reviewing and revising existing data.
- Adding new data to reflect current trends.
- Removing outdated or incorrect data.
Keeping training data fresh and accurate is vital for the reliability of AI systems. Regular updates help ensure that the AI remains effective and trustworthy.
Implementing Retrieval-Augmented Generation (RAG)
How RAG Works
Retrieval-Augmented Generation (RAG) is a method that combines document retrieval with natural language generation. This means that instead of just relying on what the AI has learned during training, it can pull in real documents to provide better answers. For example, if an AI is asked about a specific topic, it can search a database of articles or documents to find the most relevant information and use that to create a response.
Benefits of Using RAG
- Improved Accuracy: By using real documents, the AI can give more precise answers.
- Contextual Awareness: RAG helps the AI understand the context better, leading to more relevant responses.
- Reduced Hallucinations: Since the AI bases its answers on actual data, the chances of it making things up are lower.
Challenges in Implementing RAG
- Quality of Data: The effectiveness of RAG depends on the quality of the documents in the database. If the data is poor, the answers will be too.
- Search Efficiency: The AI must be able to quickly find the right documents, which can be tricky.
- Complexity of Integration: Setting up RAG can be complicated and may require technical expertise.
RAG is a powerful tool that can significantly enhance the reliability of AI systems by grounding their responses in factual data.
In summary, RAG is an innovative approach that helps AI systems provide better answers by using real documents, making them more reliable and less prone to hallucinations.
Aspect | Description |
---|---|
Method | Combines retrieval and generation |
Main Benefit | Reduces hallucinations |
Key Challenge | Ensuring data quality and efficient search |
Monitoring and Auditing AI Systems
Continuous Monitoring Techniques
To ensure AI systems are functioning correctly, continuous monitoring is essential. Here are some techniques:
- Real-time data tracking: Keep an eye on how the AI is performing as it processes information.
- Feedback loops: Use user feedback to improve the AI’s responses over time.
- Anomaly detection: Identify unusual patterns that may indicate hallucinations.
Audit Processes for AI Systems
Regular audits help maintain the reliability of AI systems. Key steps include:
- Data review: Check the quality and relevance of the training data.
- Performance evaluation: Assess how well the AI is meeting its goals.
- Compliance checks: Ensure the AI adheres to ethical standards and regulations.
Tools for Detecting Hallucinations
Several tools can help identify when AI systems are hallucinating. Some of the top artificial intelligence (AI) hallucination detection tools include:
- Semantic entropy detection: This method measures the meaning behind AI responses to spot inaccuracies.
- Predictive measurement: This technique predicts when hallucinations might occur based on past data.
- Bias detection tools: These tools help identify and correct biases in the AI’s training data.
Regular monitoring and auditing are crucial for maintaining the trustworthiness of AI systems. By implementing these practices, organizations can significantly reduce the risks associated with AI hallucinations.
Future Directions in Hallucination Reduction
Emerging Research and Techniques
The field of AI is constantly evolving, and researchers are exploring new methods to tackle hallucinations. Some promising areas include:
- Predictive models that can identify potential hallucinations before they occur.
- Advanced algorithms that enhance the accuracy of AI responses.
- Techniques that improve the understanding of context in AI-generated content.
Collaborations Between Academia and Industry
Partnerships between universities and tech companies are crucial for advancing AI reliability. These collaborations can lead to:
- Shared resources for research and development.
- Access to diverse datasets that improve training quality.
- Joint projects that focus on real-world applications of AI.
Potential of AI in High-Stakes Settings
As AI systems are deployed in critical areas like healthcare and law, ensuring their reliability becomes even more important. Future directions may include:
- Developing strict guidelines for AI usage in sensitive fields.
- Implementing robust monitoring systems to catch errors in real-time.
- Training AI models with high-quality data to minimize risks.
The journey to reduce AI hallucinations is ongoing, but with innovative techniques and collaborative efforts, we can enhance the reliability of AI systems significantly.
In summary, the future of hallucination reduction in AI looks promising, with emerging research, strong partnerships, and a focus on high-stakes applications paving the way for more reliable AI technologies.
Conclusion
In summary, using generative AI can really help businesses work better, improve experiences for customers and employees, and speed up important tasks. It’s important to know what hallucinations are, why they happen, and how to reduce them. This knowledge will make you a smarter user of AI tools. Stay tuned for the next part of our series, where we will discuss explainability. If you missed the first part, be sure to check it out!
Frequently Asked Questions
What are AI hallucinations?
AI hallucinations happen when artificial intelligence gives incorrect or made-up information. It’s like when someone confidently tells a story that isn’t true.
Why do AI systems hallucinate?
AI can hallucinate for several reasons, like using bad training data or not understanding the question properly. Sometimes, it just guesses wrong.
How can we reduce AI hallucinations?
We can lower hallucinations by using better training data, checking AI answers against trusted sources, and using special techniques to measure accuracy.
What is Retrieval-Augmented Generation (RAG)?
RAG is a method where AI first searches a reliable database for answers before responding. This helps prevent it from making mistakes.
How do we monitor AI for hallucinations?
We can keep an eye on AI by regularly checking its responses, using tools that spot errors, and running audits to find mistakes.
What are the future trends in reducing AI hallucinations?
Future efforts will focus on new research, teamwork between schools and companies, and finding ways to make AI safer for important tasks.