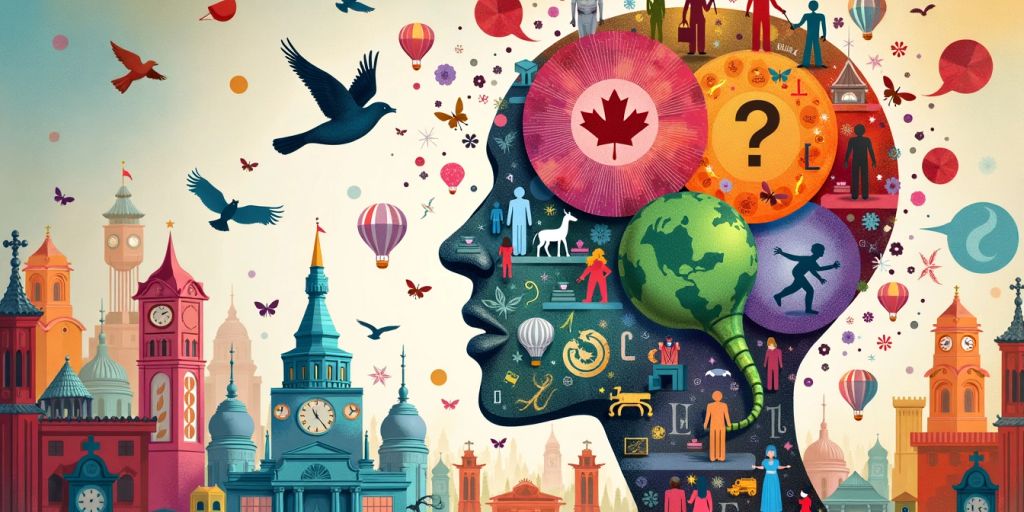
The Massive Multi-Task Language Understanding (MMLU) benchmark is a key tool in the field of artificial intelligence. It helps researchers and developers test how well their AI models understand and process language across many different tasks. This guide will explore what MMLU is, why it matters, how it works, and its real-world uses, as well as the challenges it faces.
Key Takeaways
- MMLU is designed to evaluate AI models on multiple language tasks at once.
- It helps standardize how AI models are tested, making comparisons easier.
- The benchmark encourages improvements in AI language understanding.
- MMLU has real-world applications in various industries, enhancing AI capabilities.
- There are challenges in using MMLU, including the need for significant resources and complex setups.
Understanding the MMLU Benchmark
Definition and Purpose
The MMLU benchmark, or Massive Multi-Task Language Understanding, is a tool used to evaluate how well AI models understand language across many tasks at once. It helps researchers see how effective different language processing methods are. This benchmark is important for improving AI’s ability to understand and work with human language.
Historical Background
The MMLU benchmark was created because there was a need for a single way to measure how well different AI models understand language. As AI technology grew, it became clear that we needed a better way to evaluate models on various tasks like text classification and question-answering. This led to the development of MMLU, which aims to provide a complete assessment of language understanding abilities.
Core Components
The MMLU benchmark includes several key parts:
- Task Variety: It covers a wide range of language tasks, from simple to complex.
- Scalability: It can be used for different types of AI models, making it flexible.
- Performance Metrics: It provides clear ways to measure how well models perform on language tasks.
The MMLU benchmark is a significant step forward in understanding how AI can process and interpret human language, paving the way for future advancements in technology.
Significance of the MMLU Benchmark in AI
The MMLU benchmark plays a crucial role in the field of AI and natural language processing. It serves as a key driver for innovation and improvement in language understanding abilities. By offering a standardized way to evaluate AI models across various language tasks, the MMLU benchmark helps ensure fair comparisons and encourages healthy competition among developers.
Driving Innovation
- The MMLU benchmark encourages the development of better AI models.
- It helps researchers identify strengths and weaknesses in their models.
- By providing a clear evaluation framework, it inspires new ideas and approaches in AI.
Standardization in Evaluation
- The benchmark creates a common ground for assessing AI performance.
- It allows for consistent comparisons across different models and tasks.
- This standardization helps in understanding the progress in AI technologies over time.
Impact on AI Development
- The MMLU benchmark democratizes access to AI evaluation, allowing more researchers to participate.
- It fosters collaboration and knowledge sharing among AI practitioners.
- By validating models against state-of-the-art capabilities, it pushes the boundaries of what AI can achieve.
The MMLU benchmark is not just a tool; it is a catalyst for growth in the AI community, paving the way for more advanced and capable language understanding systems.
Aspect | Description |
---|---|
Evaluation Type | Multi-task language understanding |
Number of Subjects | Covers 57 subjects, providing a broad assessment of language capabilities. |
Accessibility | Open-source framework, promoting widespread use and collaboration. |
How the MMLU Benchmark Works
Evaluation Process
The MMLU benchmark evaluates AI models through a structured process that includes several key steps:
- Task Selection: Choose the specific language tasks to assess, ensuring they align with the MMLU framework.
- Model Benchmarking: Select AI models that are capable of multi-task language understanding and evaluate them against the MMLU.
- Performance Analysis: Analyze how well the models perform on the selected tasks, identifying strengths and weaknesses.
- Iterative Refinement: Use the evaluation results to improve the models continuously.
Task Categories
MMLU covers a wide range of task categories, including:
- Text classification
- Question answering
- Language translation
- Sentiment analysis
This variety allows for a comprehensive assessment of language understanding capabilities.
Performance Metrics
To measure performance, MMLU uses various metrics, such as:
- Accuracy: The percentage of correct answers.
- F1 Score: A balance between precision and recall.
- Exact Match: The proportion of answers that exactly match the expected output.
Metric | Description |
---|---|
Accuracy | Correct answers out of total |
F1 Score | Harmonic mean of precision and recall |
Exact Match | Percentage of exact matches |
The MMLU benchmark is essential for measuring and assessing a model’s performance across key tasks. It provides a unified platform for evaluating AI models, fostering innovation in natural language processing.
Real-World Applications of the MMLU Benchmark
Case Studies
The MMLU benchmark has been applied in various real-world scenarios, showcasing its versatility and effectiveness. Here are some notable examples:
- Healthcare: AI models are evaluated for their ability to understand medical texts, aiding in diagnosis and treatment recommendations.
- Legal: The benchmark helps assess models that interpret legal documents, ensuring they can handle complex language and terminology.
- Education: AI tools are tested for their capability to understand and generate educational content, enhancing learning experiences.
Industry Adoption
Many industries are embracing the MMLU benchmark to improve their AI systems. Some key sectors include:
- Finance: For analyzing market trends and generating reports.
- Retail: To enhance customer service through chatbots and recommendation systems.
- Technology: For developing smarter virtual assistants that can understand user queries better.
Future Prospects
The future of the MMLU benchmark looks promising as it continues to evolve. Innovations in AI will likely lead to:
- More refined evaluation methods.
- Broader task categories to cover emerging language challenges.
- Enhanced collaboration between researchers and industries to push the boundaries of language understanding.
The MMLU benchmark is not just a tool; it is a catalyst for progress in AI, driving advancements across various fields.
In summary, the MMLU benchmark is crucial for assessing AI models in real-world applications, ensuring they meet the demands of diverse industries and contribute to ongoing advancements in technology.
Challenges and Limitations of the MMLU Benchmark
Implementation Complexity
Implementing the MMLU benchmark can be quite challenging. Here are some key points to consider:
- Resource Intensive: It requires significant computational power.
- Diverse Tasks: The variety of tasks can complicate the setup.
- Data Sourcing: Finding quality data for all tasks is not easy.
Scalability Issues
As the demand for AI models grows, so do the challenges related to scalability:
- Increased Data Volume: More tasks mean more data to process.
- Model Adaptation: Models need to adapt to new tasks, which can be difficult.
- Performance Variability: Different tasks may yield inconsistent results.
Resource Requirements
The MMLU benchmark demands a lot of resources, which can limit its accessibility:
- High Costs: Running extensive evaluations can be expensive.
- Technical Expertise: Requires skilled personnel to manage the benchmarks.
- Time Consumption: Evaluating models can take a long time.
The MMLU benchmark is a powerful tool, but it comes with its own set of challenges that can hinder its effectiveness in practical applications. Understanding these limitations is crucial for effective implementation.
Comparing MMLU with Other Benchmarks
GLUE and SuperGLUE
The MMLU benchmark stands out when compared to other benchmarks like GLUE and SuperGLUE. Here’s how they differ:
Feature | MMLU | GLUE | SuperGLUE |
---|---|---|---|
Task Variety | Multi-task | Single-task | Multi-task |
Difficulty Level | High | Moderate | High |
Evaluation Scope | Comprehensive | Limited | Comprehensive |
Single-Task vs Multi-Task
When we look at single-task benchmarks, they focus on one specific area, while MMLU evaluates multiple tasks at once. This multi-task approach allows for:
- Broader assessment of language understanding.
- Better comparison across different AI models.
- Identification of strengths and weaknesses in various tasks.
Advantages of MMLU
MMLU offers several benefits over traditional benchmarks:
- Holistic Evaluation: It assesses models on a wide range of tasks, providing a complete picture of their capabilities.
- Standardized Metrics: MMLU uses consistent metrics, making it easier to compare results across different models.
- Adaptability: It can be applied to various natural language processing tasks, ensuring relevance in diverse applications.
The MMLU benchmark is essential for understanding how well AI models perform across different tasks, making it a key tool in the field of language understanding.
Enhancing AI Models Using the MMLU Benchmark
Iterative Refinement
To improve AI models using the MMLU benchmark, follow these steps:
- Task Selection: Choose specific language tasks that align with the MMLU framework.
- Model Benchmarking: Test various AI models against the MMLU to see how well they perform.
- Performance Analysis: Look at the results to find strengths and weaknesses in the models.
- Iterative Refinement: Use the insights gained to make continuous improvements to the models.
Performance Optimization
Optimizing performance involves:
- Regularly updating models with new data.
- Using diverse datasets to enhance learning.
- Collaborating with other researchers to share knowledge and techniques.
Collaborative Efforts
Collaboration can lead to better outcomes. Here are some ways to work together:
- Share findings with peers to foster innovation.
- Participate in workshops and conferences to learn from others.
- Engage in joint projects to tackle complex challenges.
The MMLU benchmark is a powerful tool that helps AI developers understand how well their models are doing and where they can improve. By using it effectively, we can create smarter AI systems that better understand language.
Conclusion
In summary, the Massive Multi-Task Language Understanding (MMLU) benchmark is a groundbreaking tool in the field of artificial intelligence and language processing. It plays a crucial role in improving how machines understand human language, encouraging teamwork and new ideas. As technology progresses, the MMLU benchmark will continue to guide significant changes, helping to develop smarter AI systems that can better understand and interpret our language. This is an essential step toward creating advanced AI applications that can serve us in various ways.
Frequently Asked Questions
What is the MMLU benchmark?
The MMLU benchmark, or Massive Multi-Task Language Understanding, is a tool used to test how well AI models can understand language across many different tasks at once.
Why is the MMLU benchmark important?
It’s important because it helps improve AI by providing a standard way to measure how well models understand language, which leads to better technology.
How does the MMLU benchmark work?
It works by evaluating AI models on various tasks like answering questions and understanding text, giving a complete view of their language skills.
What are the challenges of using the MMLU benchmark?
Some challenges include needing a lot of computer power, dealing with complex tasks, and ensuring the data used is ethical and fair.
How does MMLU compare to other benchmarks?
MMLU is different because it tests models on many tasks at the same time, while other benchmarks often focus on just one task.
Can the MMLU benchmark be used for different language tasks?
Yes, it can be adapted for various language tasks, making it flexible for different types of evaluations.