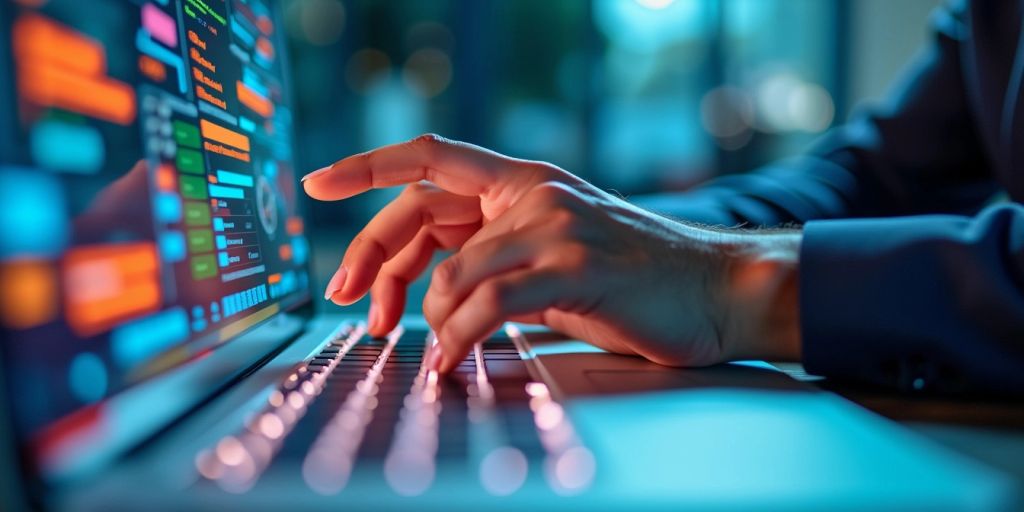
Natural Language Processing (NLP) is a key area in artificial intelligence that helps computers understand and work with human language. This guide will break down the important techniques and uses of NLP, making it easier to grasp how it shapes our daily interactions with technology.
Key Takeaways
- NLP helps machines understand and generate human language.
- Techniques like tokenization and named entity recognition are crucial for processing text.
- Applications of NLP include chatbots, sentiment analysis, and machine translation.
- Advanced models like transformers significantly enhance NLP capabilities.
- Ethical issues, such as bias and privacy, are important to consider in NLP development.
Understanding Natural Language Tasks
Defining Natural Language Processing
Natural Language Processing (NLP) is a field of artificial intelligence that helps computers understand and work with human language. It allows machines to read, interpret, and generate text in a way that is meaningful to people. This technology is used in many everyday applications, from chatbots to search engines.
Core Components of NLP
NLP consists of several key parts:
- Tokenization: Breaking text into smaller pieces, like words or sentences.
- Parsing: Analyzing the structure of sentences to understand their meaning.
- Semantic Analysis: Understanding the meaning behind words and phrases.
Challenges in NLP
Despite its advancements, NLP faces several challenges:
- Ambiguity: Words can have multiple meanings, making it hard for machines to understand context.
- Bias: Models can reflect biases present in the training data, leading to unfair outcomes.
- Complexity of Language: Human language is full of nuances, slang, and idioms that can confuse machines.
NLP is a powerful tool, but it is still evolving. As technology improves, so will our ability to communicate with machines.
Key Techniques in Natural Language Processing
Tokenization Methods
Tokenization is the first step in processing text. It involves breaking down sentences into smaller parts called tokens, which can be words or phrases. This technique is essential for many NLP tasks. Common methods include:
- Word Tokenization: Splitting text into individual words.
- Sentence Tokenization: Dividing text into sentences.
- Character Tokenization: Breaking text into individual characters.
Named Entity Recognition
Named Entity Recognition (NER) helps identify and categorize important entities in text, such as names of people, places, and organizations. This process is crucial for:
- Extracting information from large texts.
- Linking entities to databases.
- Building knowledge graphs.
Part-of-Speech Tagging
Part-of-Speech (POS) Tagging assigns grammatical labels to words in a sentence, indicating their roles. This technique is important for:
- Understanding sentence structure.
- Improving grammar checking.
- Enhancing semantic analysis.
Word Embeddings
Word embeddings are a way to represent words as numbers in a high-dimensional space. This helps computers understand the meaning of words based on their context. Techniques like Word2Vec and GloVe are commonly used. Word embeddings are vital for:
- Language modeling.
- Document classification.
- Machine translation.
Understanding these techniques is key to mastering natural language processing (NLP). They form the foundation for building effective NLP applications, such as chatbots and virtual assistants.
Applications of Natural Language Tasks
Natural Language Processing (NLP) is used in many areas of our daily lives. Here are some key applications:
Chatbots and Virtual Assistants
Chatbots and virtual assistants are among the most popular uses of NLP. These smart systems can:
- Understand user questions.
- Provide helpful answers.
- Simulate conversations like a human.
They improve customer service and make interactions easier for users.
Sentiment Analysis
Sentiment analysis helps businesses understand how people feel about their products or services. It involves:
- Analyzing customer reviews.
- Identifying positive, negative, or neutral feelings.
- Making decisions based on this feedback.
This technique allows companies to adjust their strategies to better meet customer needs.
Text Summarization
Text summarization is about shortening long texts while keeping the main ideas. This is useful for:
- News articles.
- Research papers.
- Product reviews.
It saves time for readers by providing quick insights.
Machine Translation
Machine translation automatically translates text from one language to another. This technology:
- Helps break down language barriers.
- Improves communication worldwide.
- Uses advanced models like Google Translate for better accuracy.
NLP is changing how we interact with technology, making it more accessible and efficient.
Advanced Models and Architectures
Recurrent Neural Networks
Recurrent Neural Networks (RNNs) are a type of neural network designed for processing sequences of data. They are particularly useful for tasks like language modeling and speech recognition. RNNs can remember previous inputs, which helps them understand context better than traditional models. However, they can struggle with long sequences due to issues like vanishing gradients.
Transformer Models
Transformers have changed the game in Natural Language Processing. Unlike RNNs, they use a mechanism called self-attention to process all words in a sentence at once. This allows them to capture relationships between words more effectively. The introduction of transformers has led to the development of many powerful models, including BERT and GPT-3. Transformers are now the backbone of most NLP tasks.
Large Language Models
Large Language Models (LLMs) are built on transformer architectures and trained on vast amounts of text data. They can generate human-like text and perform various tasks, such as translation and summarization. These models have raised questions about their environmental impact due to the energy required for training.
Model Name | Parameters (Billions) | Key Features |
---|---|---|
GPT-3 | 175 | Text generation, conversation |
BERT | 0.34 | Contextual understanding |
T5 | 11 | Text-to-text framework |
Future Trends in NLP
The future of NLP is bright, with ongoing research into more efficient models and applications. Innovations like Mixture of Experts (MoE) aim to improve performance by using different parameters for different tasks. As we continue to explore these advanced architectures, we can expect even more powerful and efficient NLP solutions.
The journey of mastering NLP is ongoing, with each new model bringing us closer to understanding and generating human language effectively.
Practical Implementation of NLP Solutions
Preprocessing Text Data
To effectively work with text data, preprocessing is essential. This step helps clean and prepare the data for analysis. Here are some common preprocessing techniques:
- Lowercasing: Convert all text to lowercase to ensure uniformity.
- Removing Punctuation: Eliminate punctuation marks to focus on words.
- Tokenization: Split text into individual words or phrases.
Building NLP Models with Python
Python is a popular language for NLP due to its simplicity and powerful libraries. Here’s how to get started:
- Choose a Library: Use libraries like NLTK, spaCy, or Hugging Face.
- Load Your Data: Import your text data into your Python environment.
- Train Your Model: Use algorithms to train your model on the data.
Leveraging NLP Frameworks
Frameworks can simplify the development of NLP applications. Some widely used frameworks include:
- TensorFlow: Great for building deep learning models.
- PyTorch: Known for its flexibility and ease of use.
- Hugging Face: Offers pre-trained models for quick implementation.
Evaluating NLP Models
Evaluating the performance of your NLP models is crucial. Here are some metrics to consider:
Metric | Description |
---|---|
Accuracy | Measures the percentage of correct predictions. |
Precision | Indicates the correctness of positive predictions. |
Recall | Measures the ability to find all relevant instances. |
Remember: Continuous evaluation helps improve model performance and ensures reliability in real-world applications.
In summary, mastering the practical aspects of NLP involves understanding preprocessing, utilizing Python libraries, leveraging frameworks, and evaluating models effectively. By following these steps, you can create robust NLP solutions that meet various needs.
Ethical Considerations in NLP
Bias in NLP Models
Bias in natural language processing (NLP) models is a significant concern. These models can unintentionally learn and replicate biases present in their training data. This can lead to unfair outcomes in applications like hiring or law enforcement. Here are some key points to consider:
- Models may reflect societal biases, such as gender or racial stereotypes.
- Biased outputs can harm individuals and communities.
- Continuous monitoring and updating of models are essential to mitigate bias.
Privacy Concerns
Privacy is another critical issue in NLP. As these systems process vast amounts of personal data, they can inadvertently expose sensitive information. Important considerations include:
- Ensuring data is anonymized before use.
- Implementing strong data protection measures.
- Being transparent about data usage and storage.
Environmental Impact of Training Models
Training large NLP models requires significant computational resources, leading to a considerable environmental footprint. Some points to note:
- High energy consumption contributes to greenhouse gas emissions.
- Researchers are exploring more efficient algorithms to reduce this impact.
- Sustainable practices in AI development are becoming increasingly important.
Understanding these ethical considerations is vital for anyone working in NLP. Responsible practices can help ensure that technology benefits everyone without causing harm.
Future Directions in Natural Language Tasks
Innovations in NLP
The field of Natural Language Processing (NLP) is constantly evolving. New techniques and tools are being developed to improve how machines understand and generate human language. Some exciting innovations include:
- Contextual Understanding: Enhancing models to grasp the context better, leading to more accurate responses.
- Multimodal Learning: Combining text with images and sounds to create richer interactions.
- Real-time Processing: Developing systems that can analyze and respond to language in real-time.
Integration with Other Technologies
NLP is not just a standalone field; it is increasingly being integrated with other technologies. This integration can lead to:
- Enhanced User Experiences: By combining NLP with virtual reality, users can interact in more immersive ways.
- Improved Data Analysis: Merging NLP with big data analytics helps businesses gain deeper insights from customer feedback.
- Smart Automation: Integrating NLP with robotics can lead to more intelligent and responsive machines.
Expanding Applications of NLP
The applications of NLP are growing rapidly. Here are some areas where NLP is making a significant impact:
- Healthcare: Analyzing patient records to improve care and outcomes.
- Education: Creating personalized learning experiences through intelligent tutoring systems.
- Finance: Automating customer service and fraud detection processes.
As NLP continues to advance, it holds the potential to transform industries and improve everyday life. Natural language processing can transform feedback data into actionable insights and enhance customer experiences that drive strong business revenue.
Conclusion
In summary, mastering Natural Language Processing (NLP) is essential in today’s tech-driven world. This guide has shown how NLP helps machines understand and use human language in many ways, from chatbots to translating languages. As you dive into this field, start with the basics like math and coding in Python. Online courses can be a great help. As you learn more, focus on advanced topics like neural networks and tools such as PyTorch. While NLP offers exciting opportunities, it also comes with challenges, like bias in language models. Being aware of these issues is important for anyone working in this area. Overall, NLP is a fascinating field that can make a big difference in our lives.
Frequently Asked Questions
What is Natural Language Processing (NLP)?
Natural Language Processing (NLP) is a field of study that focuses on how computers can understand and work with human language. It combines computer science and linguistics to help machines interpret text and speech.
What are some common uses of NLP?
NLP is used in many ways, such as chatbots that assist customers, tools that analyze feelings in text, and software that translates languages automatically.
What are some challenges faced in NLP?
Some challenges in NLP include understanding slang, dealing with different languages, and avoiding biases in the data used to train models.
How does tokenization work in NLP?
Tokenization is the process of breaking down text into smaller parts, like words or sentences. This helps computers analyze and understand the text better.
What is sentiment analysis?
Sentiment analysis is a technique used to determine if the feelings expressed in a piece of text are positive, negative, or neutral. It’s often used to understand customer opinions.
Why is it important to consider ethics in NLP?
Ethics in NLP is important because models can sometimes reflect biases found in their training data. This can lead to unfair treatment or misunderstandings, so it’s crucial to create fair and responsible AI.